This article has been reviewed according to Science X's editorial process and policies. Editors have highlighted the following attributes while ensuring the content's credibility:
fact-checked
peer-reviewed publication
trusted source
proofread
Machine learning model helps doctors predict opioid prescription risk for patients
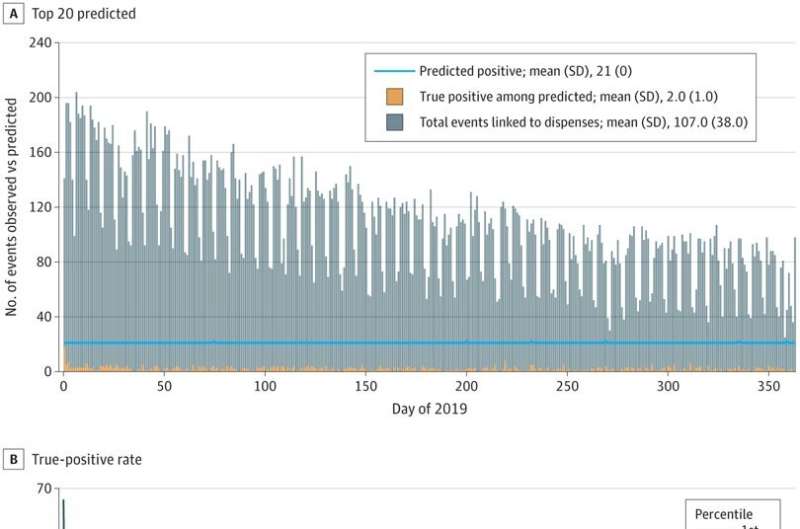
Researchers at the University of Alberta are using a form of artificial intelligence to help doctors better predict which patients are at risk of adverse outcomes from opioid prescriptions.
In newly published research, the collaborative team created a machine learning model to estimate the risk of an emergency department visit, hospitalization or death within 30 days of filling an opioid prescription. Machine learning allows computers to find patterns in huge volumes of data, getting more accurate over time as they continually validate and retrain with updated information.
The model cross-referenced pharmacy data with administrative health data—the records created every time a patient interacts with the health-care system, from emergency department and doctor visits to blood tests and scans. They tracked anonymized records for 853,324 adult Albertans in 2018 and 2019, when 6,181,025 opioid prescriptions were filled and 77,326 adverse events were reported.
Doctors follow guidelines to determine which patients are at higher risk but they don't catch everyone because they often have limited information, according to Dean Eurich, an epidemiologist and professor in the School of Public Health.
"Machine learning can expand the number of variables that we're going to look at from a couple dozen to 5,000, so we can rely on your labs, your hospitalizations, your physician visits, medications you have taken in the past—all that information can now be put into the model to predict where you are in the spectrum in terms of a low risk or a high risk of having a poor outcome," Eurich explains.
"The human mind just can't process that many pieces of information at once."
Saving lives, saving money
The model was created in partnership with health information company Okaki and the College of Physicians and Surgeons of Alberta, who in their role as administrators of the Tracked Prescription Program are responsible for daily monitoring of opioid prescriptions, one of many efforts being made to slow the ongoing opioid crisis that took 1346 Albertans' lives in 2022.
The model predicted adverse patient outcomes with 90% accuracy, Eurich reports. He expects to take the next step for the research within six months by implementing and testing the model in real time as part of the college's surveillance system.
Doctors with higher-risk patients will be notified so they can choose to prescribe a different drug, give a smaller dose or follow up with the patient more closely.
"This is a tool to help clinicians to manage their patients who are very complex and to help patients get better outcomes from their therapies," Eurich says.
Eurich is also using machine learning programs to answer other population health questions by analyzing health data, including why people with diabetes were more likely to acquire COVID-19 than the general population and why some wound up in intensive care while others did not.
"Alberta has some of the best health data sets in the world, and so we want to make sure that we're using them to their fullest potential," Eurich says.
"That really gives us the best chance to change outcomes for patients, by learning from the health system itself and putting interventions in place."
The paper is published in the journal JAMA Network Open.
More information: Vishal Sharma et al, Development and Validation of a Machine Learning Model to Estimate Risk of Adverse Outcomes Within 30 Days of Opioid Dispensation, JAMA Network Open (2022). DOI: 10.1001/jamanetworkopen.2022.48559